The Importance of AI Annotation Tools in Modern Software Development
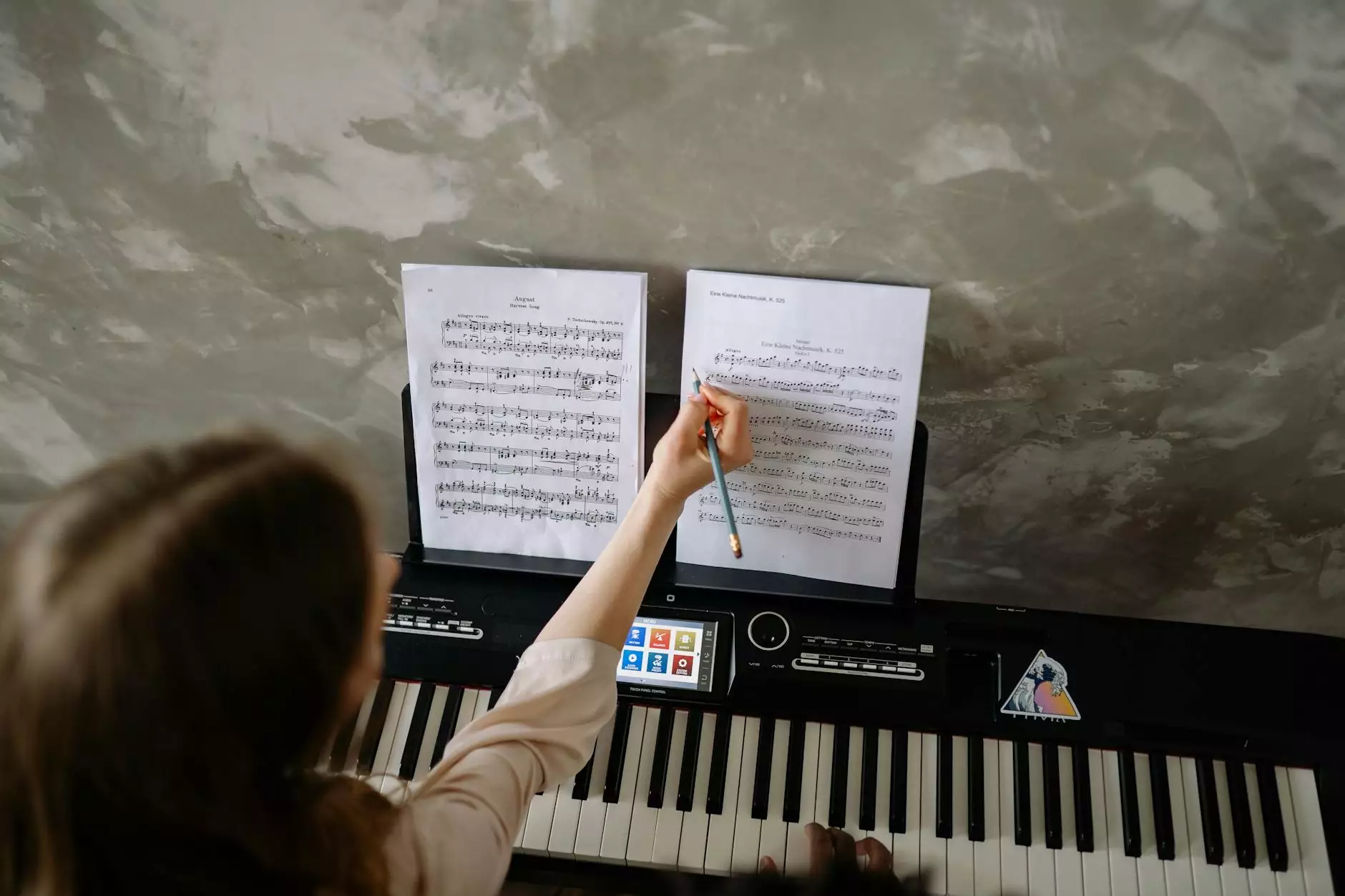
In today’s fast-paced digital world, the demand for high-quality data is greater than ever. Businesses across various sectors are leveraging the power of artificial intelligence (AI) to gain insights, improve efficiencies, and enhance decision-making processes. However, the success of AI systems heavily depends on the quality of the data fed into them. This is where AI annotation tools come into play, providing essential support in the realm of software development and data analysis.
Understanding AI Annotation Tools
AI annotation tools are software solutions designed to label and categorize data, making it easier for machine learning algorithms to understand and process information. These tools are crucial in transforming raw data into formats that are usable for training AI models. Effective data annotation ensures that AI applications operate at optimal performance and reliability.
Types of Data That Need Annotation
Businesses often work with various types of data that require annotation, including:
- Text Data: Sentiment analysis, entity recognition, and classification rely on annotated text data.
- Image Data: Image segmentation and object detection tasks require labeling different components within images.
- Audio Data: Speech recognition models benefit from transcribed audio datasets.
- Video Data: Video annotation helps in training algorithms for surveillance, driverless cars, and more.
Benefits of Using AI Annotation Tools
Integrating an AI annotation tool into your software development workflow can yield numerous benefits:
1. Enhanced Data Quality
Quality data is the backbone of successful AI projects. By utilizing robust annotation tools, businesses can improve the quality of the annotated data, reducing errors and inconsistencies. High-quality datasets lead to reliable AI models, which ultimately enhance the performance of applications.
2. Increased Efficiency
Manual data annotation is time-consuming and prone to human errors. AI annotation tools automate many of these processes, resulting in significant time savings. This allows software developers to focus on more critical aspects of their projects, such as coding and improving functionalities.
3. Scalability
As your business grows, so does the need for more data. AI annotation tools are designed to handle large volumes of data effectively, ensuring that your projects can scale without compromising quality. This scalability is essential for maintaining competitive advantage in a rapidly evolving market.
4. Cost-Effectiveness
Investing in AI annotation tools can lead to substantial cost savings. By reducing the need for extensive manual labor and increasing accuracy, organizations can minimize their operational costs associated with data management and model training.
Choosing the Right AI Annotation Tool
With various AI annotation tools available on the market, selecting the right one can be a daunting task. Here are some critical factors to consider during your decision-making process:
1. Supported Data Types
Ensure that the chosen tool supports the specific types of data you are working with, whether it be text, images, audio, or video.
2. Ease of Use
User-friendly interfaces are crucial for maximizing productivity. Look for tools that offer intuitive designs and comprehensive user support.
3. Customization Options
Different projects have different requirements. The ability to customize annotation processes is vital for ensuring that the tool meets your specific needs.
4. Integration Capabilities
Your AI annotation tool should seamlessly integrate with your existing tech stack, allowing for a smoother workflow in your software development process.
Best Practices for AI Annotation
To maximize the effectiveness of your AI annotation process, consider the following best practices:
1. Set Clear Annotation Guidelines
Well-defined guidelines are essential for maintaining consistency in data annotations. Providing specific instructions helps reduce ambiguity and variations among different annotators.
2. Utilize a Diverse Annotation Team
Involving a diverse group of annotators ensures multiple perspectives are applied, enhancing the quality and richness of the annotations provided.
3. Regular Quality Checks
Implement consistent quality assurance processes to evaluate the accuracy and reliability of annotations. Regular audits can help identify areas for improvement.
4. Continuous Feedback Loop
Creating a feedback loop allows annotators to learn from previous mistakes and improve their performance over time, leading to better data quality.
Case Studies of Successful AI Annotation Implementation
Several organizations have successfully harnessed the power of AI annotation tools to enhance their software development processes:
1. Healthcare Sector
In the healthcare industry, AI annotation tools have been used to analyze patient data and medical images. Companies that implemented these tools experienced improved diagnostic accuracy and faster patient outcomes.
2. Autonomous Vehicles
Leading companies in the automotive sector utilized AI annotation tools to label and classify thousands of hours of driving footage. This annotation is critical for enabling safe and reliable autonomous driving systems.
3. E-Commerce Platforms
E-commerce businesses leverage AI annotation tools for product classification and customer sentiment analysis. Accurate annotations help enhance search functionality and improve customer experiences.
Conclusion: The Future of AI Annotation in Software Development
As businesses continue to embrace digital transformation, the role of AI annotation tools in software development will only become more significant. The ability to streamline data processing, enhance model training, and improve overall operational efficiency makes these tools indispensable in today's competitive landscape. By investing in the right annotation solutions and adhering to best practices, companies can unlock the full potential of their data, driving innovation and success in their respective industries.
For organizations looking to embed advanced AI capabilities into their software development processes, exploring options like Keymakr's AI annotation tool is a crucial step towards ensuring data integrity and maximizing the value of AI investments.