Unlocking the Potential of Agriculture Datasets for Machine Learning
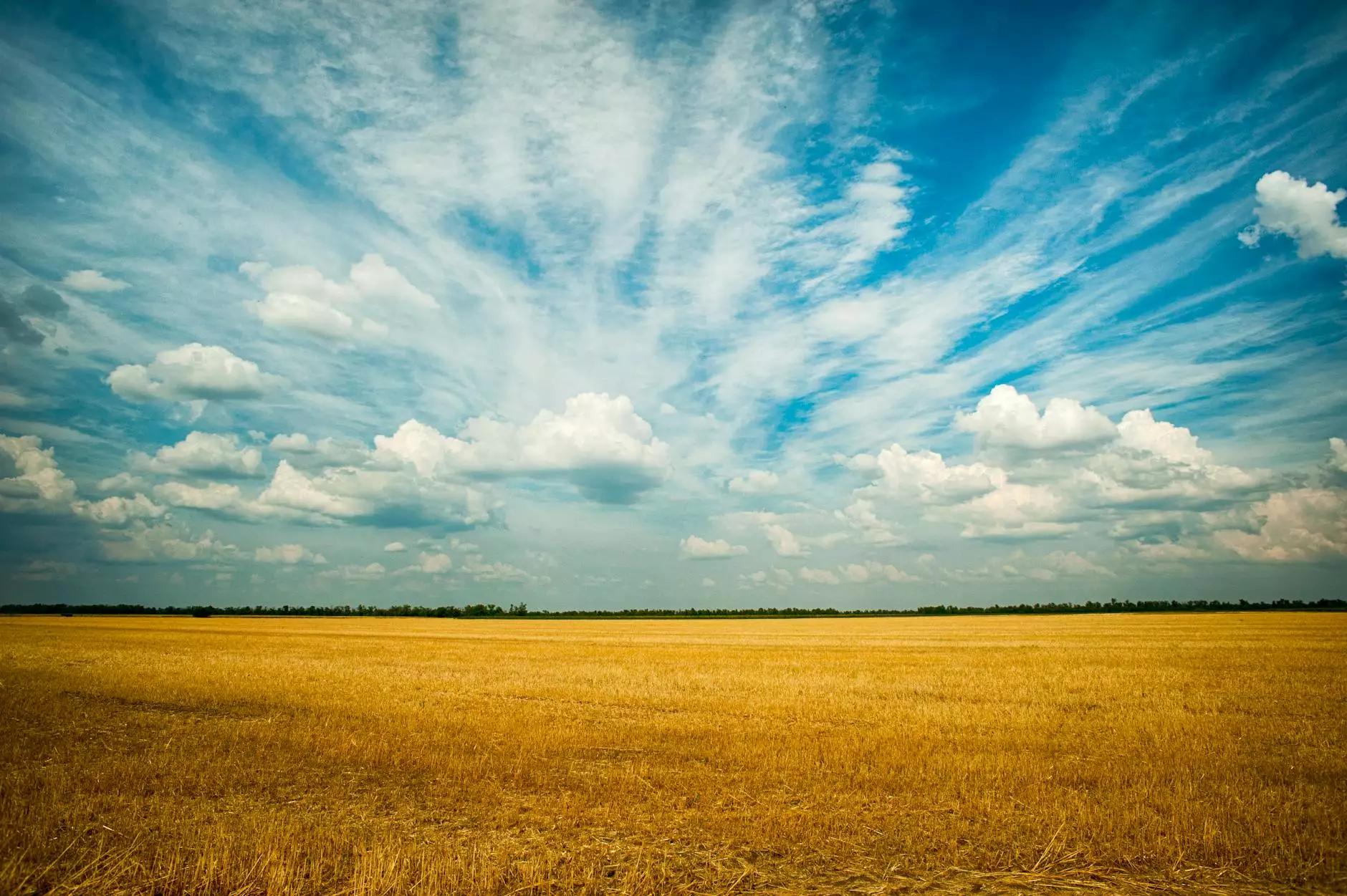
The integration of machine learning into the agricultural sector is revolutionizing the way we approach farming and food production. As we advance into an era dominated by data, the importance of utilizing agriculture datasets for machine learning cannot be overstated. These datasets provide the foundation for developing robust models that can lead to improved crop yields, efficient resource management, and enhanced sustainability. In this article, we will delve deep into the significance of agriculture datasets, the various applications of machine learning in agriculture, and how businesses can leverage these technologies for growth and innovation.
Understanding Agriculture Datasets
At its core, an agriculture dataset consists of structured and unstructured data related to farming practices, crop growth patterns, soil health, climate conditions, and market dynamics. These datasets are critical for machine learning algorithms to analyze trends and make informed predictions. Common types of agriculture datasets include:
- Soil Data: Information about soil composition, pH levels, moisture content, and nutrients.
- Weather Data: Historical and real-time climate data including temperature, precipitation, and wind speed.
- Crop Yield Data: Historical data on crop production and yields based on various factors.
- Pest and Disease Data: Information on pest populations and disease outbreaks affecting crops.
- Market Data: Prices and demand trends for various agricultural products.
The Role of Machine Learning in Agriculture
Machine learning leverages the power of algorithms to learn from data and make predictions or identify patterns without being explicitly programmed. In agriculture, machine learning can be applied in several transformative ways:
1. Precision Farming
Precision farming refers to the practice of using detailed data analysis to optimize agricultural processes. Machine learning algorithms can analyze data from sensors deployed in fields to inform farmers about the precise amounts of water, fertilizers, and pesticides needed. This not only maximizes crop yields but also minimizes waste and environmental impact.
2. Crop Disease Prediction
By utilizing agriculture datasets for machine learning, scientists can develop predictive models that identify disease outbreaks before they happen. By analyzing historical data on climate and disease patterns, farmers can effectively allocate resources and implement preventive measures.
3. Yield Prediction
Yield prediction models are another crucial application of machine learning in agriculture. These models utilize various data inputs such as weather conditions, soil health, and crop variety to predict how much yield a farmer can expect. This allows for better planning and market strategy development.
4. Supply Chain Optimization
Machine learning can greatly enhance supply chain management in agriculture. By analyzing market data and consumer trends, businesses can adjust their operations to meet demand more effectively, ensuring that they are both efficient and profitable.
Benefits of Utilizing Agriculture Datasets for Machine Learning
Leveraging agriculture datasets for machine learning presents numerous advantages for businesses in the agricultural sector:
- Improved Decision-Making: Data-driven insights lead to better decisions regarding planting, harvesting, and resource management.
- Increased Efficiency: Automating processes and using precision techniques can save time and reduce costs.
- Risk Management: Predictive models help mitigate risks associated with climate, pest outbreaks, and market fluctuations.
- Sustainability: Optimizing resource use contributes to sustainable farming practices that preserve the environment.
Challenges in Using Agriculture Datasets
While the benefits are significant, several challenges accompany the use of agriculture datasets and machine learning:
1. Data Quality and Availability
The accuracy of machine learning models largely depends on the quality of the input data. In agriculture, datasets may be incomplete, outdated, or inconsistent. Ensuring robust data collection practices is vital.
2. Integration with Existing Systems
Many farms utilize various technologies and systems that may not be compatible with machine learning tools. Integration strategies must be developed to harmonize data across different platforms.
3. Skilled Workforce
There is a growing need for skilled professionals who understand both agriculture and data science. Training programs and educational initiatives are essential to build a talent pool capable of leveraging these advanced technologies.
Case Studies: Successful Implementations
Real-world examples of successful implementations of machine learning in agriculture demonstrate its potential:
Case Study 1: The Climate Corporation
The Climate Corporation uses vast amounts of data from weather stations, satellite imagery, and soil sensors to provide farmers with precise data-driven insights. Their platform utilizes machine learning to help farmers make informed decisions that maximize yield.
Case Study 2: Blue River Technology
Blue River Technology developed a machine learning-based platform known as "See & Spray" to identify and precisely apply herbicides to crops. This innovative approach not only enhances crop health but also reduces chemical usage, promoting environmental sustainability.
Getting Started with Agriculture Datasets
For businesses looking to harness the power of agriculture datasets for machine learning, getting started involves several key steps:
1. Identify Objectives
Clearly define what you aim to achieve with machine learning. Are you looking to predict yield, optimize resource use, or enhance supply chain efficiency? Setting specific goals will guide your data collection and analysis strategies.
2. Data Collection
Invest in robust data collection methods. Utilize sensors, drones, and satellite imagery to gather high-quality data. Consider partnering with research institutions or agricultural technology companies for access to comprehensive datasets.
3. Choose the Right Tools
Select appropriate machine learning tools and frameworks that suit your business needs. Python libraries such as TensorFlow and Scikit-learn are popular choices for developing machine learning models.
4. Build Expertise
Consider hiring data scientists or consultants with expertise in agricultural analytics. Training existing staff in data analysis and machine learning methodologies can also build internal capacity.
5. Test and Iterate
Start small with pilot projects to test your models and refine them based on feedback and results. Continuously iterate on your approach to improve accuracy and efficacy.
Conclusion
In conclusion, the utilization of agriculture datasets for machine learning is transforming the agricultural landscape. By harnessing the power of data and advanced analytics, businesses can unlock new avenues for efficiency, sustainability, and growth. As technology continues to evolve, those who embrace these innovations will be better positioned to meet the challenges of modern agriculture, ensuring a prosperous future for both farmers and consumers alike.
For businesses ready to take the next step in revolutionizing their agricultural practices, leveraging keymakr.com can provide the software development services necessary to integrate advanced machine learning solutions into their operations. Together, we can cultivate a smarter, more sustainable agricultural industry.
agriculture dataset for machine learning